일 | 월 | 화 | 수 | 목 | 금 | 토 |
---|---|---|---|---|---|---|
1 | 2 | 3 | 4 | |||
5 | 6 | 7 | 8 | 9 | 10 | 11 |
12 | 13 | 14 | 15 | 16 | 17 | 18 |
19 | 20 | 21 | 22 | 23 | 24 | 25 |
26 | 27 | 28 | 29 | 30 | 31 |
- long video understanding
- q-former
- jmeter
- leetcode
- quantification
- ma-lmm
- LeNet-5
- Server
- Artificial Intelligence
- Kaggle
- 코딩테스트
- memory bank
- multimodal machine learning
- transference
- autogluon
- sliding video q-former
- CNN
- error
- tensorflow
- secure-file-priv
- Python
- hackerrank
- MySQL
- timechat
- 용어
- Anaconda
- Github
- Linux
- 백준
- timestamp-aware frame encoder
- Today
- Total
일 | 월 | 화 | 수 | 목 | 금 | 토 |
---|---|---|---|---|---|---|
1 | 2 | 3 | 4 | |||
5 | 6 | 7 | 8 | 9 | 10 | 11 |
12 | 13 | 14 | 15 | 16 | 17 | 18 |
19 | 20 | 21 | 22 | 23 | 24 | 25 |
26 | 27 | 28 | 29 | 30 | 31 |
- long video understanding
- q-former
- jmeter
- leetcode
- quantification
- ma-lmm
- LeNet-5
- Server
- Artificial Intelligence
- Kaggle
- 코딩테스트
- memory bank
- multimodal machine learning
- transference
- autogluon
- sliding video q-former
- CNN
- error
- tensorflow
- secure-file-priv
- Python
- hackerrank
- MySQL
- timechat
- 용어
- Anaconda
- Github
- Linux
- 백준
- timestamp-aware frame encoder
- Today
- Total
목록Artificial Intelligence/Paper Review (4)
Juni_DEV
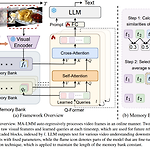
https://arxiv.org/abs/2404.05726이 논문에서는 MA-LMM(Memory-Augmented Large Multimodal Model)을 제안하여 Long-Term Video Understanding를 위한 새로운 접근법을 제시하고 있습니다. 기존의 LLM 기반 모델들이 짧은 비디오 이해에만 적합한 반면, MA-LMM은 장기 비디오 시퀀스를 온라인으로 처리하고, 이전 비디오 정보를 메모리 뱅크에 저장하여 효율적으로 분석합니다. 이로 인해 LLM의 컨텍스트 길이 제한이나 GPU 메모리 한계를 초과하지 않고도 긴 비디오를 다룰 수 있습니다.MA-LMM ArchitectureMA-LMM의 기본 구조는 크게 세 부분으로 나눌 수 있습니다.Visual Encoder: 입력된 비디오의 각 프레..
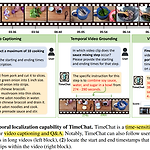
https://arxiv.org/abs/2312.02051이 논문은 긴 비디오 이해를 위해 특별히 설계된 TimeChat이라는 시간 민감형 다중모달 대형 언어 모델을 제안합니다. TimeChat은 두 가지 핵심 기능을 갖추고 있습니다. 첫째, Timestamp-aware frame encoder로 각 비디오 프레임의 시각적 콘텐츠와 타임스탬프를 결합합니다. 둘째, Sliding video Q-Former를 통해 다양한 길이의 비디오를 처리할 수 있도록 비디오 토큰 시퀀스를 생성합니다.또한, TimeChat의 명령 수행 능력을 향상시키기 위해 6개의 주요 비디오 작업을 다루는 12만 5천 개의 인스턴스를 포함한 명령 튜닝 데이터셋(TimeIT)을 구축했습니다.실험 결과, TimeChat은 제로샷 시간적 위..
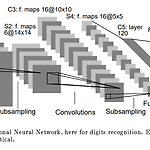
이전 글에서 pytorch로 구현해봤으니 이번에는 tensorflow를 이용해서 Lenet-5를 구현해보자구현 완료한 Tensorflow LeNet-5 Codehttps://github.com/juni5184/Paper_review/blob/main/(tensorflow)lenet-5.ipynb GitHub - juni5184/Paper_reviewContribute to juni5184/Paper_review development by creating an account on GitHub.github.com(1) 필요한 라이브러리 importimport numpy as npfrom keras.utils.np_utils import to_categoricalfrom keras.models import ..
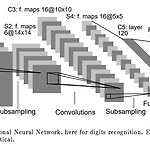
논문에 나와있는 Lenet-5 구조Input Layer : 32x32C1 (Convolution) : 28x28x6S2 (Subsampling) : 14x14x6C3 (Convolution) : 10x10x16S4 (Subsampling) : 5x5x16C5 (Fully connection) : Layer 120F6 (Fully Connection) : Layer 84Output (Gaussian connections) : 10구조까지는 파악하겠는데 도무지 어떻게 시작해야 될지 모르겠다.다른 분이 작성한 코드를 보고 Colab 이용해서 공부하는 걸로 일단 노선 변경구현 완료한 Pytorch LeNet-5 Codehttps://github.com/juni5184/Paper_review/blob/main/(..